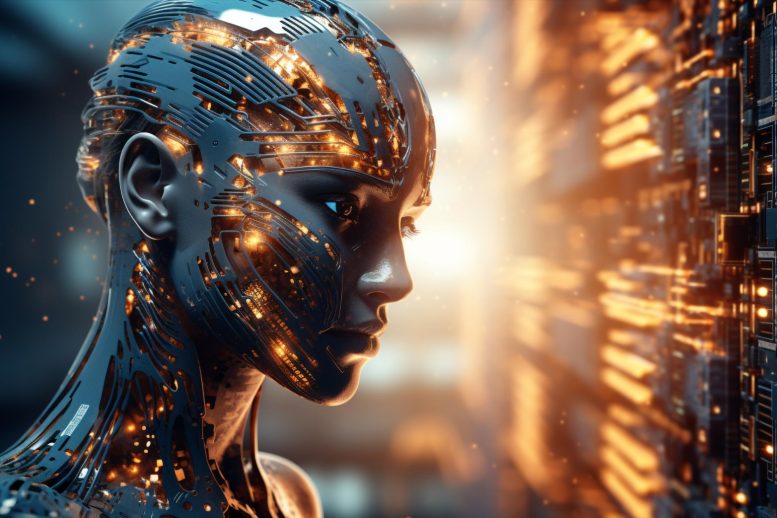
A new technique combining machine learning with traditional optimization has been shown to accelerate the solution-finding process of mixed-integer linear programming solvers by up to 70%, enhancing efficiency in logistics and other sectors. Credit: SciTechDaily.com
A new, data-driven approach could lead to better solutions for tricky optimization problems like global package routing or power grid operation.
While Santa Claus may have a magical sleigh and nine plucky reindeer to help him deliver presents, for companies like FedEx, the optimization problem of efficiently routing holiday packages is so complicated that they often employ specialized software to find a solution.
This software, called a mixed-integer linear programming (MILP) solver, splits a massive optimization problem into smaller pieces and uses generic algorithms to try and find the best solution. However, the solver could take hours — or even days — to arrive at a solution.
The process is so onerous that a company often must stop the software partway through, accepting a solution that is not ideal but the best that could be generated in a set amount of time.
Accelerating Solutions With Machine Learning
Researchers from MIT and ETH Zurich used machine learning to speed things up.
They identified a key intermediate step in MILP solvers that has so many potential solutions it takes an enormous amount of time to unravel, which slows the entire process. The researchers employed a filtering technique to simplify this step, and then used SciTechDaily