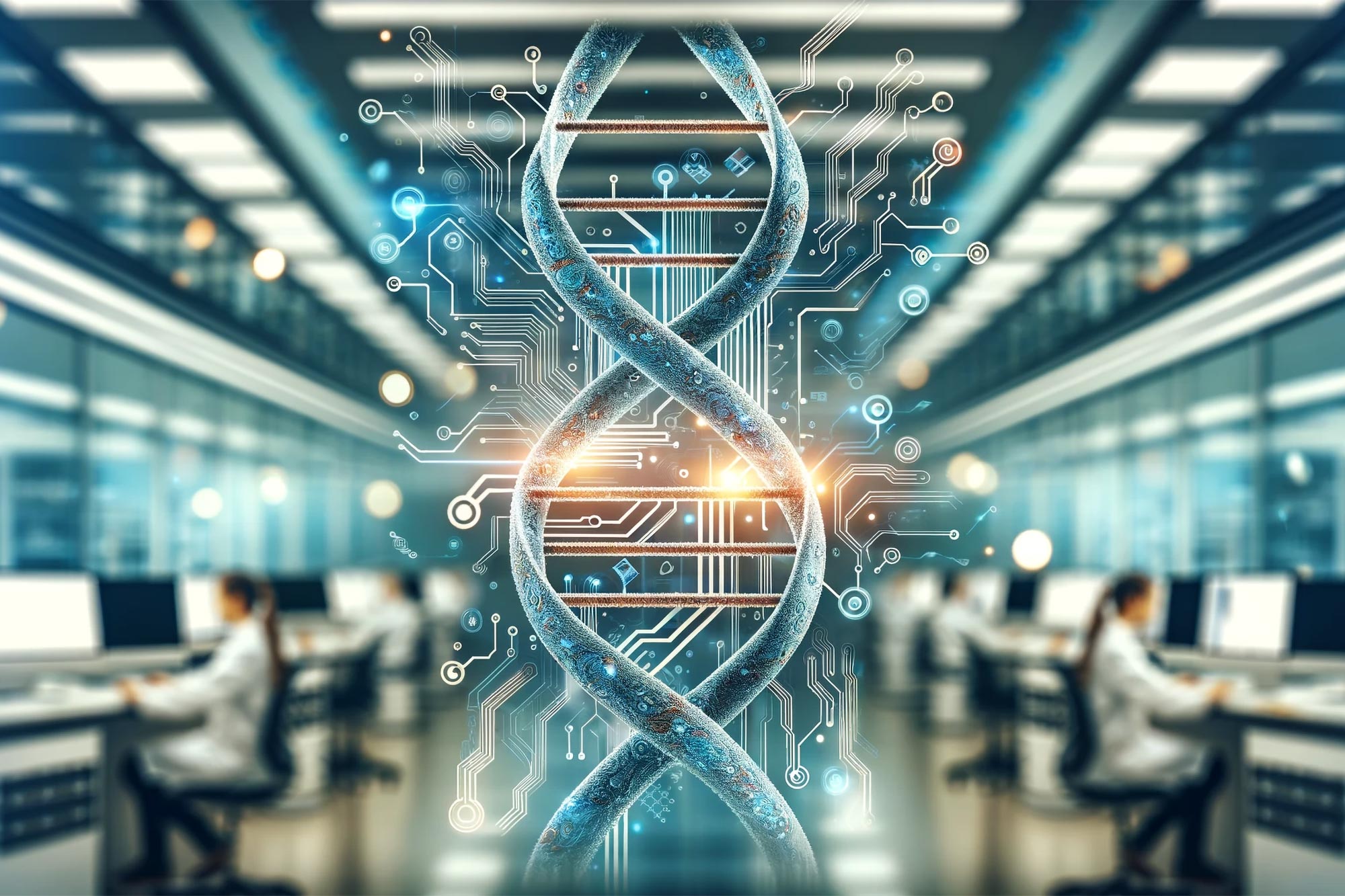
Scientists at Oak Ridge National Laboratory have advanced CRISPR Cas9 technology for microbial genome editing by using quantum biology and explainable artificial intelligence. This breakthrough allows for more precise genetic modifications in microbes, expanding the potential for renewable fuel and chemical production.
Oak Ridge National Laboratory’s research in quantum biology and AI has significantly improved the efficiency of CRISPR Cas9 genome editing in microbes, aiding in renewable energy development.
Scientists at Oak Ridge National Laboratory (ORNL) used their expertise in quantum biology, artificial intelligence, and bioengineering to improve how CRISPR Cas9 genome editing tools work on organisms like microbes that can be modified to produce renewable fuels and chemicals.
CRISPR is a powerful tool for bioengineering, used to modify genetic code to improve an organism’s performance or to correct mutations. The CRISPR Cas9 tool relies on a single, unique guide
Microbe-Focused CRISPR Research
“A lot of the CRISPR tools have been developed for mammalian cells, fruit flies, or other model species. Few have been geared towards microbes where the chromosomal structures and sizes are very different,” said Carrie Eckert, leader of the Synthetic Biology group at ORNL. “We had observed that models for designing the CRISPR Cas9 machinery behave differently when working with microbes, and this research validates what we’d known anecdotally.”
ORNL scientists developed a method that improves the accuracy of the CRISPR Cas9 gene editing tool used to modify microbes for renewable fuels and chemicals production. This research draws on the lab’s expertise in quantum biology, artificial intelligence and synthetic biology. Credit: Philip Gray/ORNL, U.S. Dept. of Energy
To improve the modeling and design of guide RNA, the ORNL scientists sought a better understanding of what’s going on at the most basic level in cell nuclei, where genetic material is stored. They turned to quantum biology, a field bridging molecular biology and quantum chemistry that investigates the effects that electronic structure can have on the chemical properties and interactions of nucleotides, the molecules that form the building blocks of
ORNL researchers validated the explainable AI model by conducting CRISPR Cas9 cutting experiments on E. coli with a large group of guides selected by the model.
Using explainable AI gave scientists an understanding of the biological mechanisms that drove results, rather than a deep learning model rooted in a “black box” algorithm that lacks interpretability, said Jaclyn Noshay, a former ORNL computational systems biologist who is first author on the paper.
“We wanted to improve our understanding of guide design rules for optimal cutting efficiency with a microbial species focus given knowledge of the incompatibility of models trained across [biological] kingdoms,” Noshay said.
The explainable AI model, with its thousands of features and iterative nature, was trained using the Summit supercomputer at ORNL’s Oak Ridge Leadership Computer Facility, or OLCF, a DOE Office of Science user facility.
Eckert said her synthetic biology team plans to work with computational science colleagues at ORNL to take what they’ve learned with the new microbial CRISPR Cas9 model and improve it further using data from lab experiments or a variety of microbial species.
Advancing CRISPR Cas9 Tools for Diverse Species
Taking quantum properties into consideration opens the door to Cas9 guide improvements for every species. “This paper even has implications across the human scale,” Eckert said. “If you’re looking at any sort of drug development, for instance, where you’re using CRISPR to target a specific region of the genome, you must have the most accurate model to predict those guides.”
Refining CRISPR Cas9 models gives scientists a higher-throughput pipeline to link genotype to phenotype, or genes to physical traits, a field known as functional genomics. The research has implications for the work of the ORNL-led Center for Bioenergy Innovation (CBI), for example, to improve bioenergy feedstock plants and bacterial fermentation of biomass.
“We’re greatly improving our predictions of guide RNA with this research,” Eckert said. “The better we understand the biological processes at play and the more data we can feed into our predictions, the better our targets will be, improving the precision and speed of our research.”
“A major goal of our research is to improve the ability to predictively modify the DNA of more organisms using CRISPR tools. This study represents an exciting advancement toward,,, understanding how we can avoid making costly ‘typos’ in an organism’s genetic code,” said ORNL’s Paul Abraham, a bioanalytical chemist who leads the DOE Genomic Science Program’s Secure Ecosystem Engineering and Design Science Focus Area, or SEED SFA, that supported the CRISPR research. “I am eager to learn how much more these predictions can improve as we generate additional training data and continue to leverage explainable AI modeling.”
Reference: “Quantum biological insights into CRISPR-Cas9 sgRNA efficiency from explainable-AI driven feature engineering” by Jaclyn M Noshay, Tyler Walker, William G Alexander, Dawn M Klingeman, Jonathon Romero, Angelica M Walker, Erica Prates, Carrie Eckert, Stephan Irle, David Kainer and Daniel A Jacobson, 20 September 2023, Nucleic Acids Research.DOI: 10.1093/nar/gkad736
Co-authors on the publication included ORNL’s William Alexander, Dawn Klingeman, Erica Prates, Carrie Eckert, Stephan Irle and Daniel Jacobson; Tyler Walker, Jonathan Romero and Angelica Walker of the Bredesen Center for Interdisciplinary Research and Graduate Education at the University of Tennessee, Knoxville; and Jaclyn Noshay and David Kainer, who were formerly with ORNL and now with Bayer and the University of Queensland, respectively.
Funding for the project was provided by the SEED SFA and CBI, both part of the DOE Office of Science Biological and Environmental Research Program, by ORNL’s Lab-Directed Research and Development program, and by the high-performance computing resources of the OLCF and Compute and Data Environment for Science, both also supported by the Office of Science.