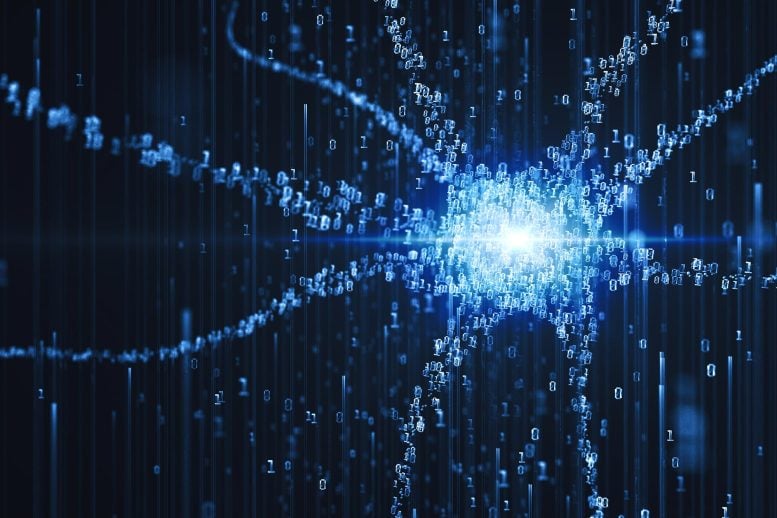
A new model developed by Flatiron Institute researchers proposes that biological neurons have more control over their surroundings than previously thought, something that could be replicated in the artificial neural networks used in machine learning.
A new model from the Flatiron Institute’s Center for Computational Neuroscience proposes that individual neurons are more influential controllers within their networks than previously believed, challenging the outdated 1960s model.
This advanced understanding could significantly enhance artificial neural network functionalities by integrating mechanisms similar to those in human brains, addressing current AI limitations such as errors and inefficient training processes.
Modern AI and Neuronal Models
Nearly all the neural networks that power modern
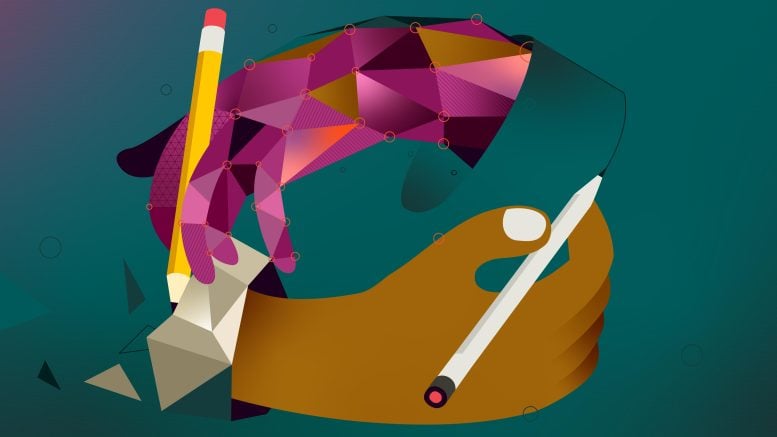
An artist’s illustration of a digital hand and a human hand drawing one another. Credit: Alex Eben Meyer for Simons Foundation
Revolutionizing AI with Advanced Neuronal Models
The new model developed at CCN posits that individual neurons exert more control over their surroundings than previously thought. The updated neuron model could ultimately lead to more powerful artificial neural networks that better capture the powers of our brains, the model developers say. The researchers present the revolutionary model in a paper published the week of June 24 in the journal Proceedings of the National Academy of Sciences.
“Neuroscience has advanced quite a bit in these past 60 years, and we now recognize that previous models of neurons are rather rudimentary,” says Dmitri Chklovskii, a group leader at the CCN and senior author of the new paper. “A neuron is a much more complex device — and much smarter — than this overly simplified model.”
The Functional Mechanism of Artificial Neural Networks
Artificial neural networks aim to mimic the way in which the human brain processes information and makes decisions, albeit in a much more simplified manner. These networks are built of ordered layers of ‘nodes’ based on the 1960s neuron model. The network starts with an input layer of nodes that receives information, then has middle layers of nodes that process the information, and then ends with an output layer of nodes that sends out the results.
Typically, a node will only pass information to the next layer if the total input it receives from the previous layer’s nodes exceeds a certain threshold. When current artificial neural networks are trained, information goes through a node in only one direction, and there’s no way for nodes to influence the information they receive from nodes earlier in the chain.
In contrast, the newly published model treats neurons as tiny ‘controllers,’ an engineering term for devices that can influence their surroundings based on information gathered about those surroundings. Not merely passive relays of input, our brain cells may actually work to control the state of their fellow neurons.
Implications and Benefits of the Neuron-As-Controller Model
Chklovskii believes that this more realistic model of a neuron-as-controller could be a significant step toward improving the performance and efficiency of many SciTechDaily