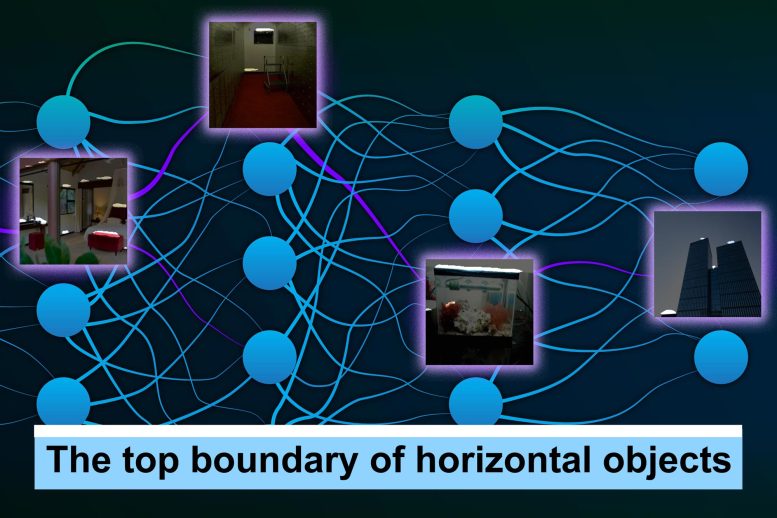
MIT researchers created a technique that can automatically describe the roles of individual neurons in a neural network with natural language. In this figure, the technique was able to identify “the top boundary of horizontal objects” in photographs, which are highlighted in white. Credit: Photographs courtesy of the researchers, edited by Jose-Luis Olivares, MIT
A new method automatically describes, in natural language, what the individual components of a neural network do.
Neural networks are sometimes called black boxes because, despite the fact that they can outperform humans on certain tasks, even the researchers who design them often don’t understand how or why they work so well. But if a neural network is used outside the lab, perhaps to classify medical images that could help diagnose heart conditions, knowing how the model works helps researchers predict how it will behave in practice.
For instance, in a neural network trained to recognize animals in images, their method might describe a certain neuron as detecting ears of foxes. Their scalable technique is able to generate more accurate and specific descriptions for individual neurons than other methods.
In a new paper, the team shows that this method can be used to audit a neural network to determine what it has learned, or even edit a network by identifying and then switching off unhelpful or incorrect neurons.
“We wanted to create a method where a machine-learning practitioner can give this system their model and it will tell them everything it knows about that model, from the perspective of the model’s neurons, in language. This helps you answer the basic question, ‘Is there something my model knows about that I would not have expected it to know?’” says Evan Hernandez, a graduate student in the MIT Computer Science and Artificial Intelligence Laboratory (CSAIL) and lead author of the paper.
Co-authors include Sarah Schwettmann, a postdoc in CSAIL; David Bau, a recent CSAIL graduate who is an incoming assistant professor of computer science at Northeastern University; Teona Bagashvili, a former visiting student in CSAIL; Antonio Torralba, the Delta Electronics Professor of Electrical Engineering and Computer Science and a member of CSAIL; and senior author Jacob Andreas, the X Consortium Assistant Professor in CSAIL. The research will be presented at the International Conference on Learning Representations.
Automatically generated descriptions
Most existing techniques that help machine-learning practitioners understand how a model works either describe the entire neural network or require researchers to identify concepts they think individual neurons could be focusing on.
The system Hernandez and his collaborators developed, dubbed MILAN (mutual-information guided linguistic annotation of neurons), improves upon these methods because it does not require a list of concepts in advance and can automatically generate natural language descriptions of all the neurons in a network. This is especially important because one neural network can contain hundreds of thousands of individual neurons.
MILAN produces descriptions of neurons in neural networks trained for computer vision tasks like object recognition and image synthesis. To describe a given neuron, the system first inspects that neuron’s behavior on thousands of images to find the set of image regions in which the neuron is most active. Next, it selects a natural language description for each neuron to maximize a quantity called pointwise mutual information between the image regions and descriptions. This encourages descriptions that capture each neuron’s distinctive role within the larger network.
“In a neural network that is trained to classify images, there are going to be tons of different neurons that detect dogs. But there are lots of different types of dogs and lots of different parts of dogs. So even though ‘dog’ might be an accurate description of a lot of these neurons, it is not very informative. We want descriptions that are very specific to what that neuron is doing. This isn’t just dogs; this is the left side of ears on German shepherds,” says Hernandez.
The team compared MILAN to other models and found that it generated richer and more accurate descriptions, but the researchers were more interested in seeing how it could assist in answering specific questions about computer vision models.
Analyzing, auditing, and editing neural networks
First, they used MILAN to analyze which neurons are most important in a neural network. They generated descriptions for every neuron and sorted them based on the words in the descriptions. They slowly removed neurons from the network to see how its