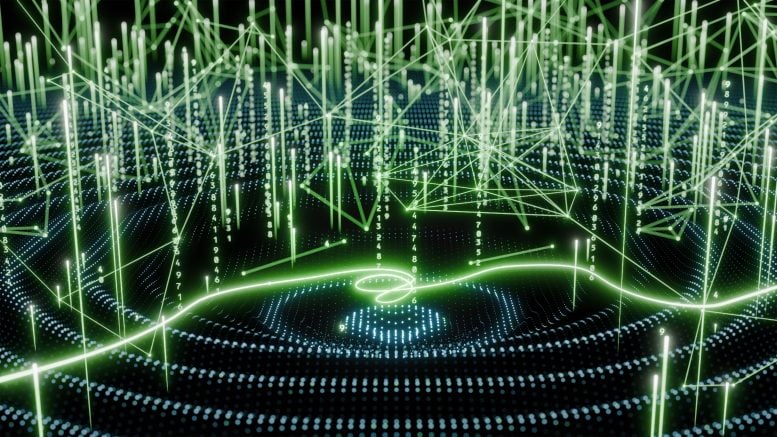
Artist’s impression of optoacoustic computing. Credit: Long Huy Da
Researchers have developed a way to use sound waves in optical neural networks, enhancing their ability to process data with high speed and energy efficiency.
Optical neural networks may provide the high-speed and large-capacity solution necessary to tackle challenging computing tasks. However, tapping their full potential will require further advances. One challenge is the reconfigurability of optical neural networks.
A research team in the Stiller Research Group at the Max Planck Institute for the Science of Light, in collaboration with the Englund Research Group at the Massachusetts Institute of Technology, has now succeeded in laying the foundation for new reconfigurable neuromorphic building blocks by adding a new dimension to photonic
The researchers use light to create temporary acoustic waves in an optical fiber. The sound waves generated in this way can for instance enable a recurrent functionality in a telecom optical fiber, which is essential to interpreting contextual information such as language.
AI and Energy Efficiency
Artificial intelligence is now commonplace and helps us juggle daily tasks. Language models such as ChatGPT are able to create naturally formulated texts, and summarize paragraphs in a structured way, thus helping us to reduce our administrative overheads. The downside is their enormous energy requirements, meaning that as they evolve, these intelligent devices will require new solutions to speed up signal processing and reduce energy consumption.
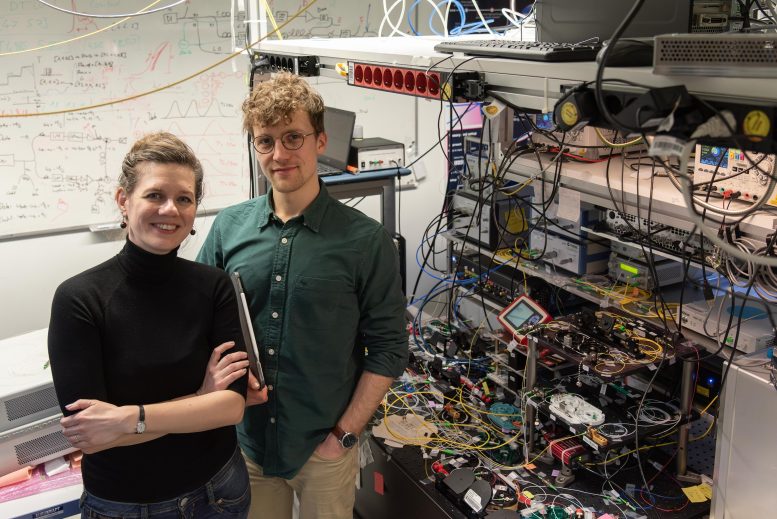
Dr. Birgit Stiller and Steven Becker in the lab. Credit: Susanne Viezens, MPL
Optical Neural Networks: A New Frontier
Neural networks have the potential to form the backbone of artificial intelligence. Building them as optical neural networks – based on light instead of electric signals – promises the handling of large volumes of data at high speeds and with great energy efficiency. To date, however, many of the experimental approaches to implementing optical neural networks have relied on fixed components and steady devices.
Now an international research team led by Birgit Stiller at the Max-Planck Institute for the Science of Light, in collaboration with Dirk Englund from Massachusetts Institute of Technology, has found a way to build reconfigurable building blocks based on sound waves for photonic machine learning. For their experimental approach, the researchers use hair-thin optical fibers, already globally used for fast internet connections.
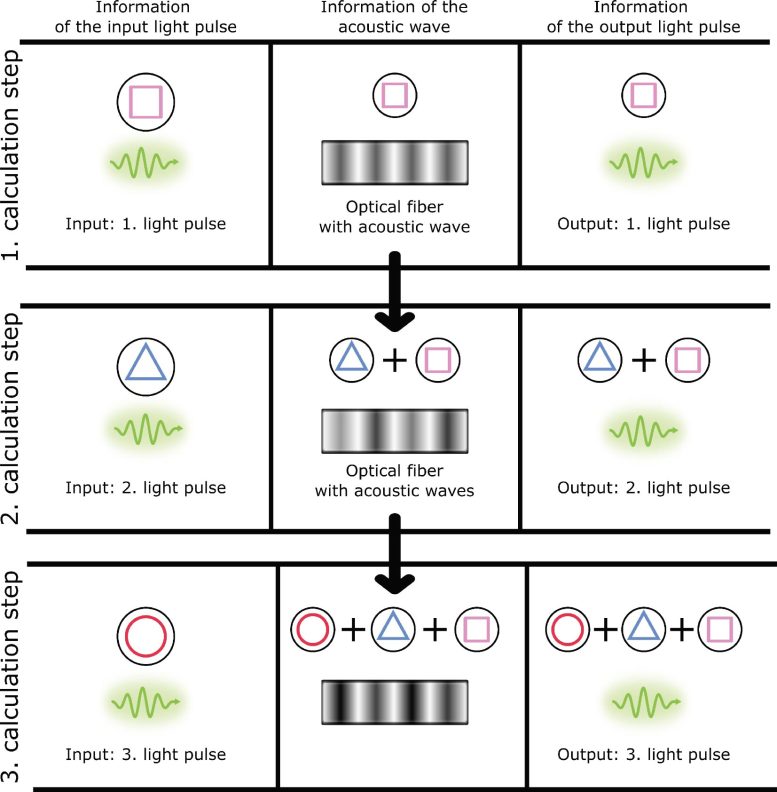
Information carried by an optical pulse is partially converted into an acoustic wave. The information remains in the acoustic wave even after the light pulse has left the optical fiber. This initial acoustic wave affects the second and third light-sound processing step with the subsequent input pulses carrying different information than the previous ones. As a result, acoustic waves connect in time seperated dynamics and serve as an information propagation medium. Credit: Stiller Research Group, MPL
Sound Waves Enhancing Optical Networks
The key to the invention is the light-driven creation of traveling sound waves that manipulate subsequent computational steps of an optical neural network. Optical information is processed and correlated to acoustic waves. The sound waves have a much longer transmission time than the optical information stream. Therefore, they remain in the optical fiber longer and can be linked to each subsequent processing step in turn. The uniqueness of this process lies in the fact that it is completely controlled by light and does not require complicated structures and transducers.
“I’m very excited that we have embarked on this new line of research pioneering the use of sound waves to control optical neural networks. Our research findings have the potential to spark the development of novel building blocks for new photonic computation architectures,” says Dr. Birgit Stiller, head of the Quantum Optoacoustics Research Group.
The first building block experimentally demonstrated by the team is a recurrent operator, a technology widely used in the field of recurrent neural networks. It allows the linking of a series of computational steps and therefore provides a context for each single calculation step performed.
Recurrent Operations in Optical Networks
In human language, for example, the order of the words can determine the meaning of a sentence. For example, the two sentences “She decided to research the challenge.” and “She decided to challenge the research.” consist of the same words but have different meanings. This is because of the different contexts created by the orders of the words. A traditional fully-connected neural network on a computer faces difficulties capturing context because it requires access to memory. In order to overcome this challenge, neural networks have been equipped with recurrent operations that enable internal memory and are capable of capturing contextual information.
Although these recurrent neural networks are straightforward to implement digitally, the analogous implementation in optics is challenging and has so far relied on artificial cavities to provide the memory.
The researchers have now used sound waves to implement a recurrent operator. As a result, the Optoacoustic REcurrent Operator (OREO) harnesses the intrinsic properties of an optical waveguide without the need for an artificial reservoir or newly fabricated structures. OREO offers the advantage of being entirely optically controlled, making the optoacoustic computer programmable on a pulse-by-pulse basis. For instance, the researchers have used this to implement a recurrent dropout optically for the first-time, a regulation technique only previously used to boost the performance of digital recurrent neural networks. OREO has been used to distinguish up-to 27 different patterns, demonstrating its ability to process context.
Future Potential of Photonic Machine Learning
“The all-optical control of OREO is a powerful feature. Especially the possibility to program the system on a pulse-by-pulse basis gives several additional degrees of freedom. Using sound waves for photonic machine learning is disrupting the status quo and I am very eager to see how the field will evolve in the future,” says Steven Becker, doctoral student in the Stiller Lab.
In the future, using sound waves for optical neural networks could unlock a new class of optical neuromorphic computing which could be reconfigured spontaneously and would allow large-scale in-memory computing in the present telecommunication network. Also, on-chip implementations of optical neural networks can benefit from this approach, which is implementable in photonic waveguides without additional electronic controls.
“Photonic machine learning might hold huge potential for parallel processing of information and energy-efficient operations. Adding acoustic waves can contribute to this endeavor with an all-optically-controlled and easy-to-operate tool-kit,” says Dr. Birgit Stiller.
Reference: “An optoacoustic field-programmable perceptron for recurrent neural networks” by Steven Becker, Dirk Englund and Birgit Stiller, 16 April 2024, SciTechDaily