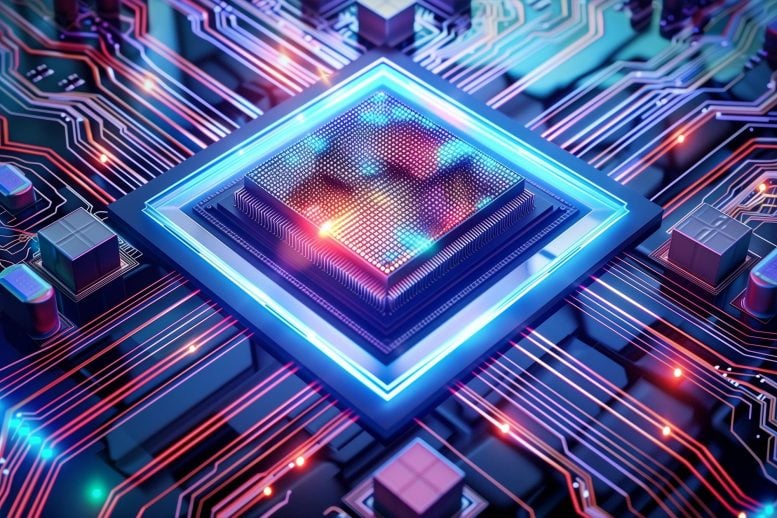
Sieun Chae from Oregon State University has pioneered a new AI chip that enhances energy efficiency by six times using a novel material system. This breakthrough aims to cut down AI’s hefty energy consumption by mimicking the integrated processing methods of biological neural networks. Credit: SciTechDaily.com
A new AI chip could potentially increase energy efficiency by six times, aligning computation and data storage in a manner akin to biological neural networks and significantly reducing AI’s electrical footprint.
A researcher from the College of Engineering at Oregon State University has contributed to the development of a new
Sieun Chae, assistant professor of electrical engineering and computer science, is working to help shrink the technology’s electricity footprint. She is researching chips, based on a novel material platform, that allows for both computation and data storage, mimicking the way biological neural networks handle information storage and processing.
Findings from her research were recently published in the journal Nature Electronics.
Efficient AI Processing
“With the emergence of AI, computers are forced to rapidly process and store large amounts of data,” Chae said. “AI chips are designed to compute tasks in memory, which minimizes the shuttling of data between memory and processor; thus, they can perform AI tasks more energy efficiently.”
The chips feature components called memristors – short for memory resistors. Most memristors are made from a simple material system composed of two elements, but the ones in this study feature a new material system known as entropy-stabilized oxides (ESOs). More than a half-dozen elements comprise the ESOs, allowing their memory capabilities to be finely tuned.
Memristors are similar to biological neural networks in that neither has an external memory source – thus no energy is lost to moving data from the inside to the outside and back. By optimizing the SciTechDaily