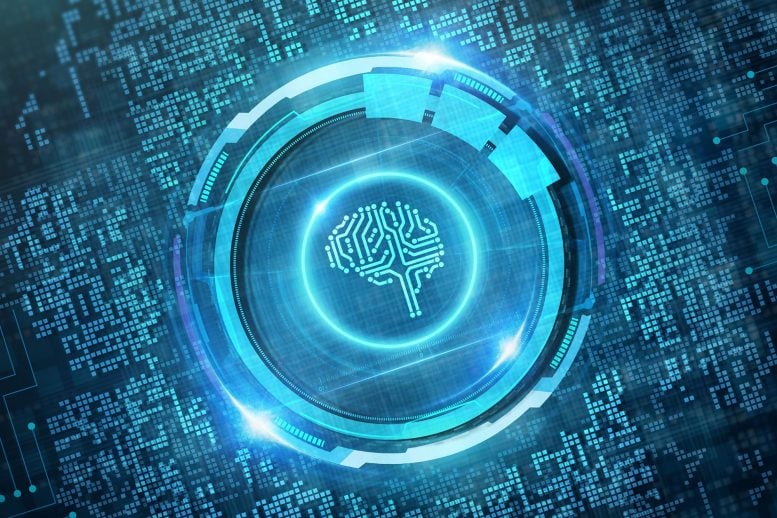
Advanced machine learning algorithms have shown potential in efficiently controlling complex systems, promising significant improvements in autonomous technology and digital infrastructure.
Recent research highlights the development of advanced
The Limitations of Linear Controllers
Many everyday devices, like thermostats and cruise control, utilize linear controllers – which use simple rules to direct a system to a desired value. Thermostats, for example, employ such rules to determine how much to heat or cool a space based on the difference between the current and desired temperatures.
Yet because of how straightforward these algorithms are, they struggle to control systems that display complex behavior, like chaos.
As a result, advanced devices like self-driving cars and aircraft often rely on machine learning-based controllers, which use intricate networks to learn the optimal control algorithm needed to best operate. However, these algorithms have significant drawbacks, the most demanding of which is that they can be extremely challenging and computationally expensive to implement.
The Impact of Efficient Digital Twins
Now, having access to an efficient digital twin is likely to have a sweeping impact on how scientists develop future autonomous technologies, said Robert Kent, lead author of the study and a graduate student in physics at The Ohio State University.
“The problem with most machine learning-based controllers is that they use a lot of energy or power and they take a long time to evaluate,” said Kent. “Developing traditional controllers for them has also been difficult because chaotic systems are extremely sensitive to small changes.”
These issues, he said, are critical in situations where milliseconds can make a difference between life and death, such as when self-driving vehicles must decide to brake to prevent an accident.
The study was published recently in SciTechDaily