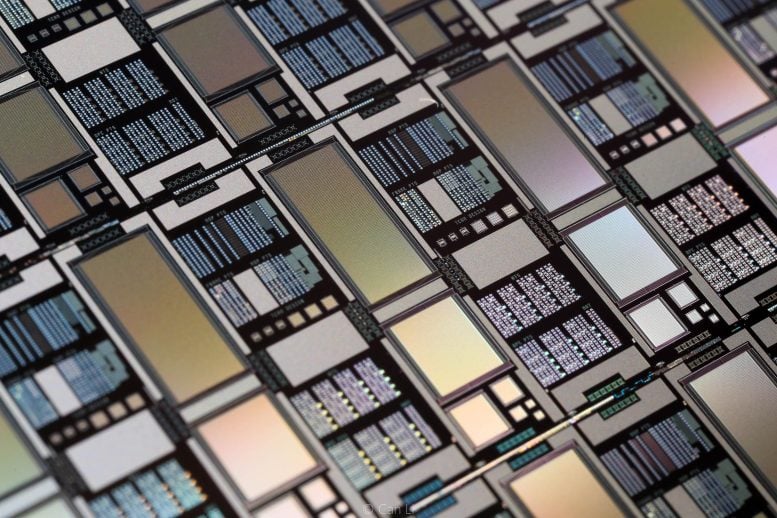
An exemplar photograph of an integrated chip containing memristor crossbar arrays of various sizes made at UMass Amherst. Credit: Can Li
UMass Amherst research demonstrates that a memristor device can solve complex scientific problems using significantly less energy, overcoming one of the major hurdles of digital computing.
A team of researchers including University of Massachusetts Amherst engineers have proven that their analog computing device, called a memristor, can complete complex, scientific computing tasks while bypassing the limitations of digital computing.
Tackling Modern Computing Challenges
Many of today’s important scientific questions—from
Qiangfei Xia, UMass Amherst professor of electrical and computer engineering, and one of the corresponding authors of the research published in the journal Science, explains that, with current computing methods, every time you want to store information or give a computer a task, it requires moving data between memory and computing units. With complex tasks moving larger amounts of data, you essentially get a processing “traffic jam” of sorts.
Revolutionizing Computing With Memristor Technology
One way traditional computing has aimed to solve this is by increasing bandwidth. Instead, Xia and his colleagues at UMass Amherst, the University of Southern California, and computing technology maker, TetraMem Inc. have implemented in-memory computing with analog memristor technology as an alternative that can avoid these bottlenecks by reducing the number of data transfers.
The team’s in-memory computing relies on an electrical component called a memristor—a combination of memory and resistor (which controls the flow of electricity in a circuit). A memristor controls the flow of electrical current in a circuit, while also “remembering” the prior state, even when the power is turned off, unlike today’s transistor-based computer chips, which can only hold information while there is power. The memristor device can be programmed into multiple resistance levels, increasing the information density in one cell.
Enhancing Computational Efficiency
When organized into a crossbar array, such a memristive circuit does analog computing by using physical laws in a massively parallel fashion, substantially accelerating matrix operation, the most frequently used but very power-hungry computation in neural networks. The computing is performed at the site of the device, rather than moving the data between memory and processing. Using the traffic analogy, Xia compares in-memory computing to the nearly empty roads seen at the height of the pandemic: “You eliminated traffic because [nearly] everybody worked from home,” he says. “We work simultaneously, but we only send the important data/results out.”
Previously, these researchers demonstrated that their memristor can complete low-precision computing tasks, like SciTechDaily