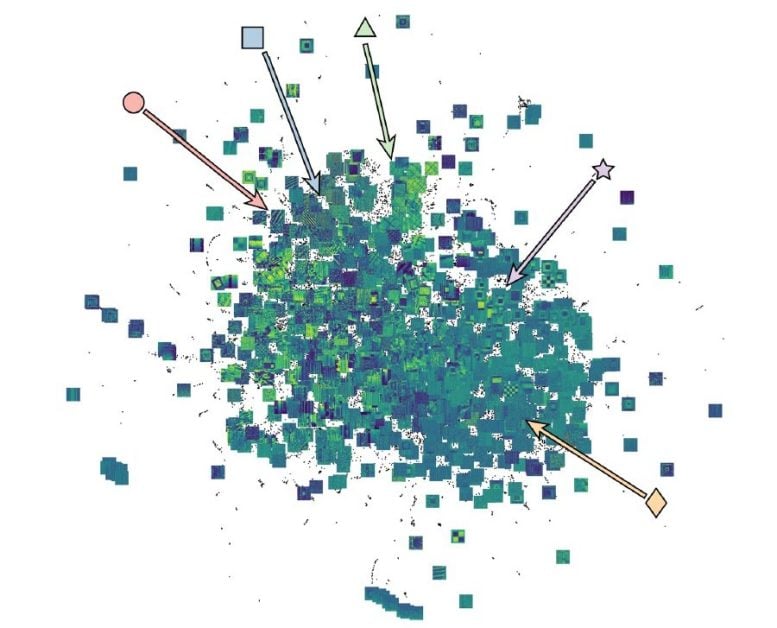
Low-dimensional uniform manifold approximation projection showing symmetry-aware image similarity from a database of greater than 25,000 piezoresponse force microscopy images. Credit: Joshua Agar/Lehigh University
A novel neural network to understand symmetry, speed materials research.
Using a large, unstructured dataset gleaned from 25,000 images, scientists demonstrate a novel machine learning technique to identify structural similarities and trends in materials for the first time.
Understanding structure-property relations is a key goal of materials research, according to Joshua Agar, a faculty member in
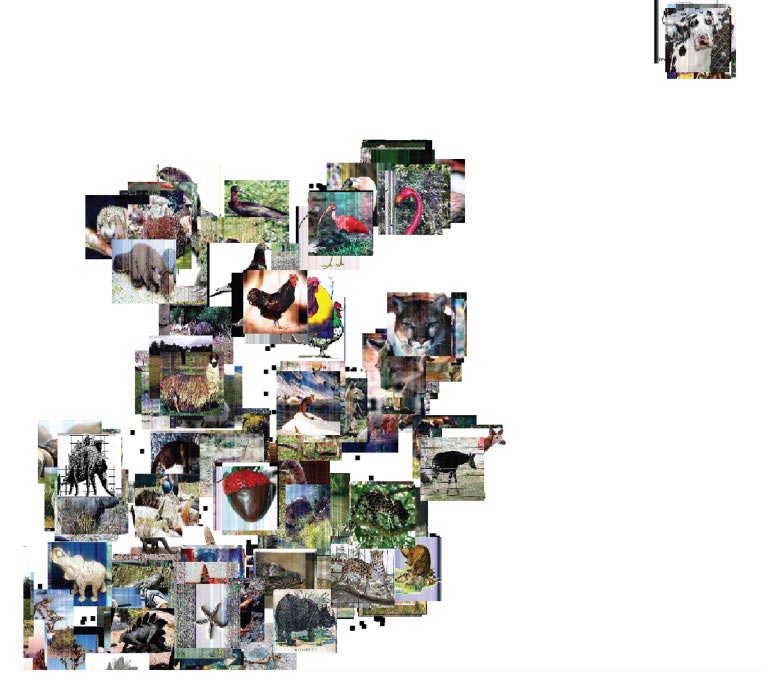
Low-dimensional uniform manifold approximation projection to visualize how neural networks learn semantic similarity of natural images. Credit: Joshua Agar/Lehigh University
Now, a team led by Lehigh University has developed a novel machine learning approach that can create similarity projections via machine learning, enabling researchers to search an unstructured image database for the first time and identify trends. Agar and his collaborators developed and trained a neural network model to include symmetry-aware features and then applied their method to a set of 25,133 piezoresponse force microscopy images collected on diverse materials systems over five years at the